Danh Cuong Tran1, Anh Linh Dang1, Thi Ngoc Lan Hoang2, Chi Thanh Nguyen3, Thi Minh Phuong Le4, Thi Ngoc Mai Dinh5, Van Anh Tran5, Thi Kim Phuong Doan2 and Thi Trang Nguyen2.
1 Center for Prenatal Diagnosis, National Hospital of Obstetrics and Gynecology, Hanoi, Vietnam.
2 Department of Biomedical and genetics, Hanoi Medical University, Hanoi, Vietnam.
3 Department of Specialized Software, Academy of Military Science and Technology, Hanoi, Vietnam.
4
Department of Basic Sciences in Medicine and Pharmacy, University of
Medicine and Pharmacy - Vietnam National University, Hanoi, Vietnam.
5 Department of Pediatrics, Hanoi Medical University, Hanoi, Vietnam.
Published: May 1, 2023
Received: January 11, 2023
Accepted: April 16, 2023
Mediterr J Hematol Infect Dis 2023, 15(1): e2023026 DOI
10.4084/MJHID.2023.026
This is an Open Access article distributed
under the terms of the Creative Commons Attribution License
(https://creativecommons.org/licenses/by-nc/4.0),
which permits unrestricted use, distribution, and reproduction in any
medium, provided the original work is properly cited.
|
Abstract
Introduction:
The prevalence of thalassemia among the Vietnamese population was
studied, and clinical decision support systems for prenatal screening
of thalassemia were created. The aim of this report was to investigate
the prevalence of thalassemia in the Vietnamese population, building a
clinical decision support system for prenatal screening for
thalassemia. Methods:
A cross-sectional study was conducted on pregnant women and their
husbands visiting the Vietnam National Hospital of Obstetrics and
Gynecology from October 2020 to December 2021. A total of 10112 medical
records of first-time pregnant women and their husbands were
collected. Results:
A clinical decision support system was built, including 2 different
types of systems for prenatal screening for thalassemia (an expert
system and 4 AI-based CDSS). One thousand nine hundred ninety-two cases
were used to train and test machine learning models, while 1555 cases
were used for specialized expert system evaluation. There were ten key
variables for AI-based CDSS for machine learning. The four most
important features in thalassemia screening were identified. The
accuracy of the expert system and AI-based CDSS was compared. The rate
of patients with Alpha thalassemia is 10.73% (1085 patients), the rate
of patients with betathalassemia is 2.24% (227 patients), and 0.29% (29
patients) of patients carry both alphathalassemia and beta-thalassemia
gene mutations. The expert system showed an accuracy of 98.45%. Among
the AI-based CDSS developed, the multilayer perceptron (MLP) model was
the most stable regardless of the training database (accuracy of 98,5%
using all features and 97% using only the four most important
features). Conclusions:
When comparing the expert system with the AI-based CDSS, the accuracy
of the expert system and AI-based models was comparable. The developed
expert system for prenatal thalassemia screening showed high accuracy.
AI-based CDSS showed satisfactory results. Further development of such
systems is promising with a view to their introduction into clinical
practice.
|
Introduction
Thalassemia is an inherited autosomal recessive disease characterized by impaired synthesis of hemoglobin protein chains.[1]
A normal mature hemoglobin molecule (HbA) consists of two pairs of
alpha and beta chains. Normal adult blood also contains ≤ 2.5%
hemoglobin A2 (HbA2) (consisting of alpha and delta chains) and <2%
hemoglobin F (HbF, fetal hemoglobin), which has gamma chains instead of
beta chains.[2] Thalassemia is caused by a gene
mutation of the gene responsible for globin chain synthesis, based on
which alpha-thalassemia and, beta-thalassemia, delta-beta-thalassemia
are distinguished.[3] Such a gene can be inherited
from one parent or two. The child's body produces fewer or no
hemoglobin chains. The production of the other chains that make up
globin does not end. As a result, unstable protein components are
produced that destroy the blood cells.[4] Thalassemia
is thus the result of reduced synthesis of at least one globin
polypeptide chain (beta, alpha, gamma, delta), resulting in abnormal
erythrocytes that are microcytic, often irregularly shaped, and prone
to hemolysis. This results in anemia and often in iron overload.[5]
The
severity of the disease depends on the number of mutated alleles. In
humans, the alpha chain of hemoglobin is encoded by two pairs of genes,
while the beta chain has only one pair. Patients with one alpha +
allele are clinically normal and are called asymptomatic carriers.
Heterozygotes with defects in 2 of the 4 genes (small alpha
thalassemia) tend to develop microcytic anemia of mild to moderate
severity but with a subclinical course. Defects in 3 of the four genes
significantly impair alpha-chain synthesis, in which hemolytic anemia
and splenomegaly are common. A defect in all 4 is a fatal condition
that causes intrauterine fetal death.[6] Minor
beta-thalassemia occurs in asymptomatic heterozygotes with a mild to
moderate clinical picture of microcytic anemia. Intermediate
beta-thalassemia presents a variable clinical picture due to the
inheritance of 2 beta-thalassemia alleles. Large beta-thalassemia
(Cooley's anemia) occurs in homozygous patients or complex
heterozygotes due to a severe beta-globin defect. These patients
develop severe anemia and bone marrow hyperactivity.[7,8]
In addition, it is rare to find simultaneous disorders in both alpha-
and beta-chain at once, but in this case, the disease may be milder
because there is little imbalance between the two types of chains.[3]
Thus, severe forms of the disease seriously affect physical
development, causing patients to need continuous blood transfusions for
life with many complications in the liver, heart, endocrine glands, and
bones. The disease is life-threatening, worsening the patient's quality
of life, and involves expensive treatments that burden the family and
society.[9]
Worldwide, an estimated 7% of the
population carries the thalassemia gene, and each year between 300,000
and 500,000 babies are born with severe homozygosity for the disease.[10,11]
In Vietnam, more than 5 million people carry the gene; every year, more
than 100,000 children carry the gene, and 1,700 children have the
severe disease due to mutations in both genes.[12]
Thalassemia is distributed in all provinces and ethnic groups
throughout the country, especially ethnic minorities in mountainous
provinces.[12]
Thalassemia is a preventable
disease screening pregnant women and their husbands at risk of carrying
the disease gene to prevent having children with the disease.[13,14]
Nowadays,
modern technology has been researched and applied in medicine to
support doctors in patient care and practice specialization.[15]
A Clinical Decision Support System (CDSS) is "any electronic or
non-electronic system designed to aid directly in clinical decision
making, in which characteristics of individual patients are used to
generate patient-specific assessments or recommendations that are then
presented to clinicians for consideration".[16] CDSSs are classified as expert knowledge-based systems and artificial intelligence (AI).[17]
CDSSs have many advantages, such as reducing the rate of misdiagnosis,
improving efficiency and patient care, and reducing the risk of
medication errors.[18] For thalassemia, there have
been studies around the world applying AI in screening carriers with
high efficiency. In 2002, Amendolia and colleagues[19]
studied and built a real-time classification system based on Artificial
Neural Networks (ANNs) to distinguish thalassemia gene carriers and
normal people with an accuracy of 94%, a sensitivity of 92% and a
specificity of 95%. In 2013, Masala et al.[20]
compared the performance of The Radial Basis Function (RBF) network,
The Probabilistic Neural Network (PNN), and The K-Nearest Neural
Network (KNN) algorithms in thalassemia screening with 304 data
samples. The results show that the RBF algorithm had a sensitivity of
93% and a specificity of 91%, similar to the results of KNN of 80% and
91% and PNN of 89% and 73%.[20]
The screening
for thalassemia in Vietnam is still mainly based on the two indexes
(mean corpuscular volume (MCV) and mean corpuscular hemoglobin (MCH))
and performed manually, and no CDSS has been built yet. This causes
many difficulties in disease prevention at primary health care
facilities and in ethnic minority areas because of the limited
understanding of thalassemia not only by the people but also by the
grassroots medical staff. The requirement is to build AI software and
an expert knowledge-based system for thalassemia screening that can be
applied to even primary healthcare facilities.
Thus, developing
and introducing into clinical practice modern CDSSs for screening
thalassemia in Vietnam is an urgent task. These systems can
significantly assist doctors in making optimal decisions, even in
primary healthcare institutions. Special attention should be paid to
CDSSs based on machine learning algorithms, which remain poorly studied.
Therefore, our research was carried out with the following objectives:
1. Investigating the prevalence of thalassemia in the Vietnamese population
2. Building a clinical decision support system for prenatal screening for thalassemia.
Material and Methods
Study design.
This cross-sectional study was conducted from October 2020 to December
2021. Data were collected using a convenient sampling method. We
collected data on pregnant women and their husbands when they came to
the Vietnam National Hospital of Obstetrics and Gynecology for annual
screening of congenital disabilities through medical records. Data were
collected from the medical records of patients who came to the hospital
before the study.
Ethical approval of the study.
The research was approved by the Vietnam National Hospital of
Obstetrics and Gynecology Ethics committee, Minutes 54/5 of 2020-09-12.
The research has been performed in accordance with the Declaration of
Helsinki.
Study subjects.
A total of 10112 medical records of first-time pregnant women and their
husbands were collected, of which 1992 cases were used to train and
test machine learning models while 1555 cases were used for specialized
knowledge system evaluation. All patients underwent routine screening
of thalassemia: peripheral blood smear, complete blood count (CBC),
hemoglobin quantification by high-performance liquid chromatography
(HPLC) and capillary electrophoresis (CE), and iron status tests.
Detection of hemoglobin gene mutations by polymerase chain reaction
(PCR) was performed for 1,364 patients and 658 newborns to assess the
prevalence of different forms of thalassemia in the Vietnamese
population. The multiplex ligation-dependent probe amplification (MLPA)
technique has been used for the molecular detection of
alpha-thalassemia. The Reverse Dot-Blot PCR technique has been used for
the molecular detection of beta-thalassemia.
Data analysis.
Two CDSS models for thalassemia pre-screening have been created: 4
AI-based CDSS for machine learning and an expert system. The basic
difference between these two types of systems is that one is based on
the knowledge base gathered from the knowledge of experts, and the
other is based on computer mining knowledge from medical data. The
specialized expert system was built based on the guideline for prenatal
screening for thalassemia of the Vietnamese Ministry of Health.
The
following independent variables were used in expert system CDSS: 4 most
important indicators from CBC result (according to MID and MDA
algorithms), including hemoglobin (HGB), mean corpuscular hemoglobin
(MCH), mean corpuscular volume (MCV), red blood cell distribution width
(RDW), serum ferritin concentration from iron status tests result, HbA2
and HbF levels from HPLC result. In addition, one should take into
account the history of hydrops fetalis and having children or family
members diagnosed with thalassemia.
There were 10 key variables
for AI-based CDSSs for machine learning: the dependent variable was
whether a patient had a thalassemia gene; 9 independent variables,
including 7 from the CBC result were hematocrit (HCT), mean corpuscular
hemoglobin (MCH), mean corpuscular hemoglobin concentration (MCHC),
mean corpuscular volume (MCV), hemoglobin (HGB), red blood cell
distribution width (RDW), red blood cell (RBC) and the other 2 are iron
status: serum iron and serum ferritin. In addition, AI-based CDSSs for
machine learning were evaluated using the four most important
indicators above-mentioned (according to MID and MDA algorithms).
Data
from 1992 pregnant women and their husbands were used to train and test
4 machine learning models, which were K-nearest neighbors (KNN),
Support vector machine (SVM), Random Forest (RF), and Multilayer
perceptron (MLP). The purpose of these models is the screening for
thalassemia gene in pregnant women, husbands, and both pregnant women
and husbands. Thus, we used data from all participants and divided them
into 2 subsets, 1 with data from pregnant women only and the other from
the husbands. After analyzing the dependent variable, whether the
participant had a thalassemia gene or not, we realized that there were
more participants without a thalassemia gene than those who did, which
caused an unbalance in the dataset and resulted in the inaccuracy of
all models. To solve this problem, we performed Synthetic Minority
Over-sampling Technique (SMOTE). This method was introduced in 2002 by
Nitesh Chawla et al.;[21] the idea is based on the
K-Nearest Neighbors algorithm. We get one sample randomly from the
minority layer a and one of its k nearest neighbors in the feature
space, then we randomly choose a k nearest neighbors b and draw a line
between these samples in the feature space. New samples are created on
this line as the combination of a and b. These new samples helped
balance the datasets, meaning the number of participants with the
thalassemia gene is now equal to those without this gene. Datasets were
then standardized using the z-score method; in particular, we used a
StandardScaler command from the sci-kit-learn library. Hyperparameters
were found using grid search. All 4 models were tested using 10-fold
cross-validation using these datasets and evaluated by 4 indices:
accuracy, precision, recall, and F1-score to find the best one.
Results
Prevalence of types of thalassemia.
Ten thousand one hundred twelve pregnant women and their husbands
performed the CBC, HPLC, and iron status during the study period. Based
on the CBC, HPLC, and iron status results, 1,364 pregnant women and
their husbands were prescribed a genetic test due to the suspected
presence of thalassemia genes. The genetic test resulted in 1,085
(10.73% of the total number of pregnant women and their husbands)
alpha-thalassemia cases and 227 (2.24%) beta-thalassemia cases. A small
ratio of 0.29% inherited both α– and β–thalassemia genes, and 0.23%
were others, including HbE disease, alpha thalassemia/HbE,
beta-thalassemia/HbE and hemoglobin Constant Spring disease (Table 1).
Among 658 fetuses of parents with identified thalassemia genes who
performed the genetic testing, the frequency of α-thalassemia (61.85%)
was also higher than β-thalassemia (13.07%) and others (including HbE
disease, α-thalassemia/HbE, and β-thalassemia/HbE) (0.61%) (Table 2).
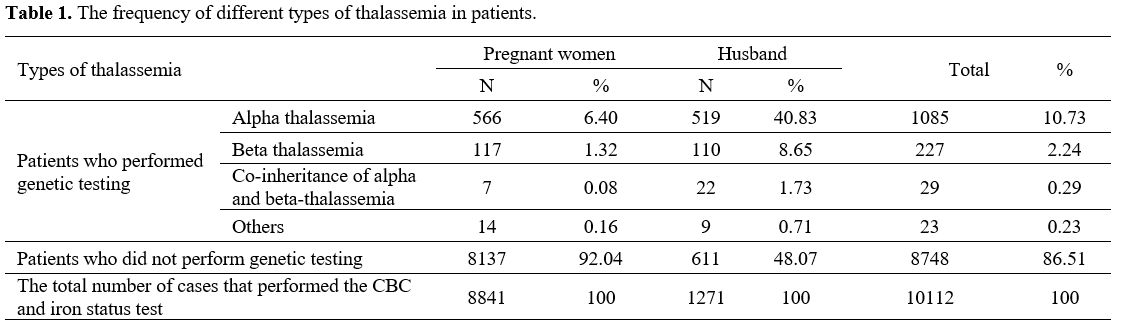 |
Table 1. The frequency of different types of thalassemia in patients. |
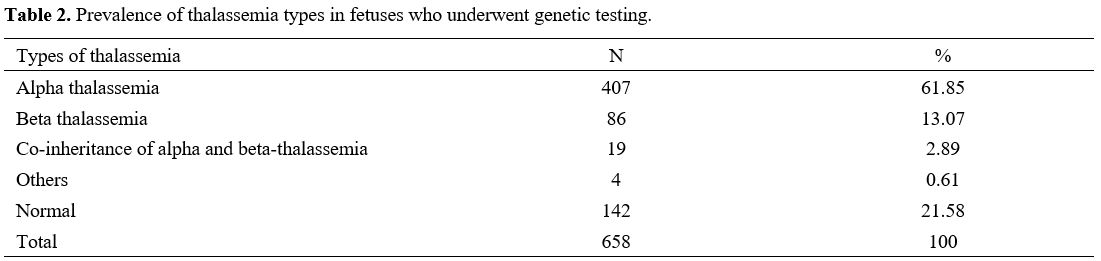 |
Table 2. Prevalence of thalassemia types in fetuses who underwent genetic testing.
|
Determining the features of the CBC, HPLC, and iron status test needed to screen for thalassemia.
After using MID and MDA algorithms, we found that 4 indices included,
HGB, MCV, MCH, and RDW were the most important ones in the screening of
thalassemia from the database containing 10112 cases having CBC, HPLC,
and iron status results (Figures 1, 2).
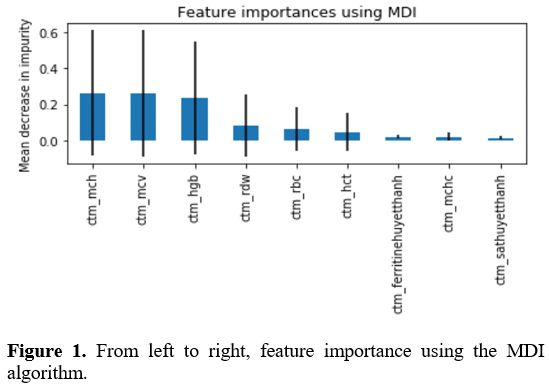 |
Figure 1. From left to right, feature importance using the MDI algorithm.
|
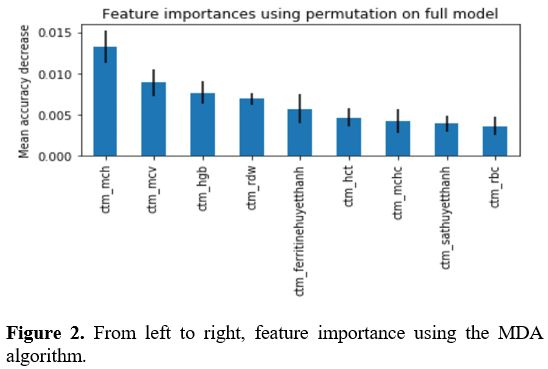 |
Figure 2. From left to right, feature importance using the MDA algorithm.
|
Selection and evaluation of the effectiveness of AI models in screening for thalassemia.
- The experimental result with general CBC and iron status database. According to Table 3,
when using the 4 most important indicators in thalassemia screening
instead of using them all in CBC and iron status database, the Accuracy
and Precision indices decreased but not much. With several models, the
result was even more improved. For example, with the SVM model,
Accuracy and Precision indices were increased (95% and 91.25% versus
94.5% and 90.12%).
KNN model using only 4 important features and
training with the general CBC and iron status database (pregnant women
and their husbands) had an accuracy of 97%, a precision of 93.75%, a
recall of 98.68%, and an F1-score of 98.15% for original data and an
accuracy of 92.5%, a precision of 85.9%, a recall of 98.68%, and an
F1-score of 90.91% for SMOTE; MLP model had an accuracy of 97%, a
precision of 92.68%, a recall of 99.23%, and an F1-score of 96.20% for
original data and an accuracy of 94.5%, a precision of 88.24%, a recall
of 98.68%, and an F1-score of 93.17% for SMOTE. SVM and RF models
showed similar but slightly lower results for some indices (Table 3).
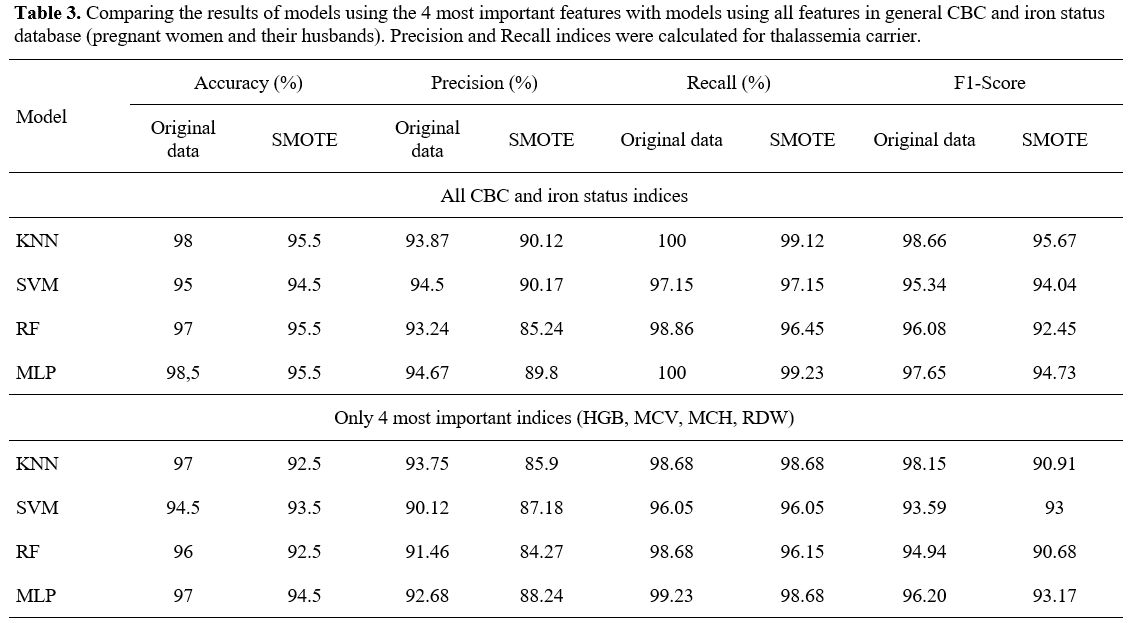 |
- Table 3. Comparing the
results of models using the 4 most important features with models using
all features in general CBC and iron status database (pregnant women
and their husbands). Precision and Recall indices were calculated for
thalassemia carrier.
|
- The experimental result with CBC and iron status database of pregnant women.
With the data filtered for pregnant women, the training and testing are
similar to the full one, including pregnant women and their husbands.
The results are shown in Table 4.
The
results showed that among the 4 training models, the KNN and MLP models
were the best with 96.93% and 96.91% accuracy, 93.22% and 93.25%
precision, 98.21% and 100% recall when using all CBC and iron status
features. Wherein, with only the 4 most important features, the
accuracy of the KNN and MLP models impressively increased from 96.93%
to 97.53% and 96.91% to 97.81%. The accuracy of the SVM model also
increased from 95.68% to 96.91% (Table 4).
One special issue with this database was that after balancing the
dataset with SMOTE technique, the result was improved for some indexes.
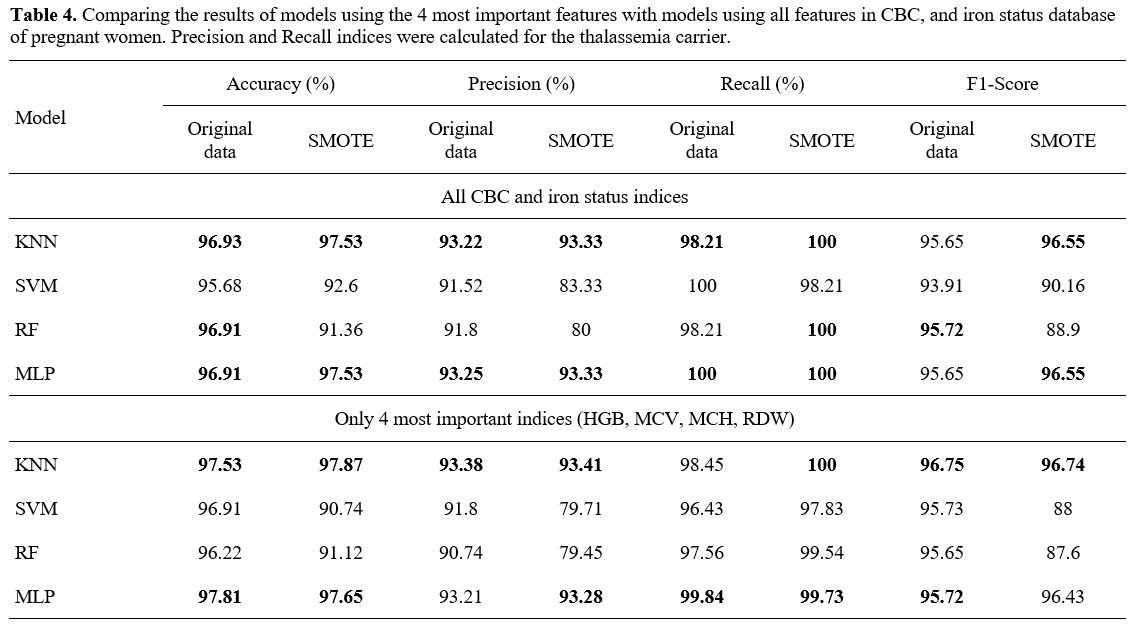 |
- Table 4. Comparing the
results of models using the 4 most important features with models using
all features in CBC, and iron status database of pregnant women.
Precision and Recall indices were calculated for the thalassemia
carrier.
|
- Experimental result with CBC and iron status database of husband.
Similar to data on complete blood counts and iron status from pregnant
women, the packaged models were not influenced by gender. With full CBC
and iron status, the RF model showed the highest accuracy at 97.55%,
precision index at 95.46%, and recall index at 100% (Table 5).
Using only 4 important features did not change the results, except for
the multilayer perceptron (MLP) model, whose accuracy was increased
significantly to 97.44%. One remarkable issue with this database was
that after balancing the dataset with SMOTE technique, the result of
the models was decreased for some indexes (Table 5).
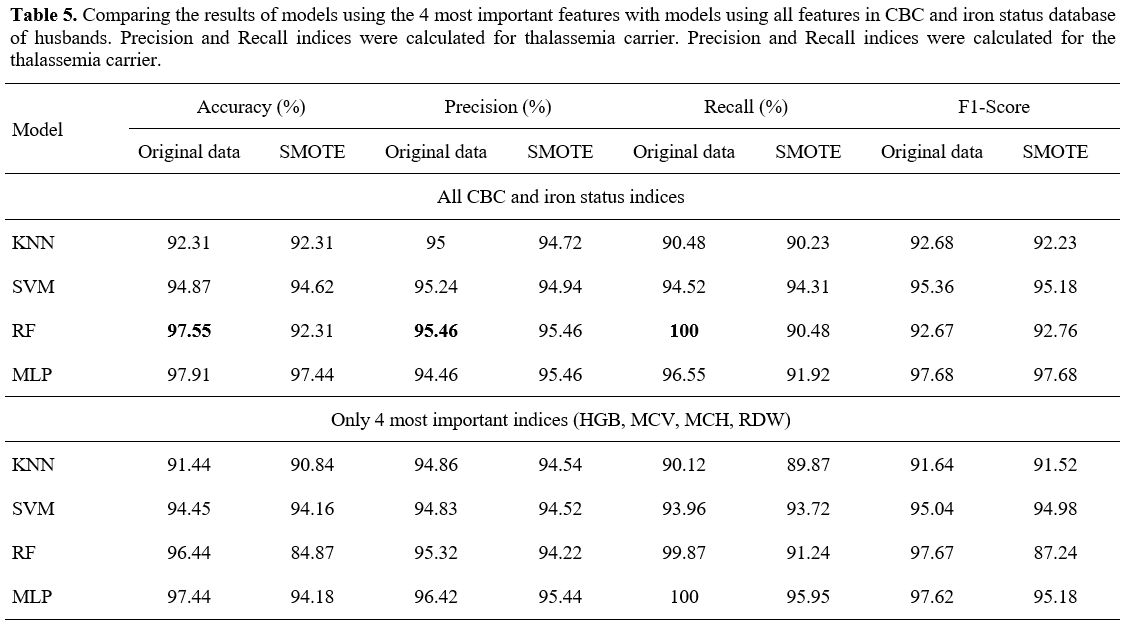 |
- Table 5. Comparing the
results of models using the 4 most important features with models using
all features in CBC and iron status database of husbands. Precision and
Recall indices were calculated for thalassemia carrier. Precision and
Recall indices were calculated for the thalassemia carrier.
|
Evaluation of the effectiveness of the expert system (ES) in screening for thalassemia and comparison with AI models. To build an expert system (ES) in screening for thalassemia, we applied the rules in Table 6.
The
effectiveness of the expert system was evaluated in the same CBC, HPLC,
and iron status database used to test the AI models, including 396
pregnant women and husbands who met the inclusion criteria (presence of
thalassemia gene by PCR). However, the result showed that 323 cases
were determined the risk of having thalassemia by the software, and 73
cases that were not. The reason for 73 cases was that patients had
performed only CBC tests, neither serum ferritin nor hemoglobin variant
analysis, and no related history, which caused a lack of indicators to
predict. 323 cases left were evaluated and calculated, shown in Tables 7 and 8.
There were 5 incorrect cases in risk assessment (false positive) and no
false negative cases, which meant no thalassemia patients were left
out. Comparing the expert system with the AI model, the accuracy of the
expert system was 98.45%, while that of the KNN and MLP models was 97%,
the RF model – 96%, and the SVM model – 94,5% when using only the 4
most important features (Table 8)
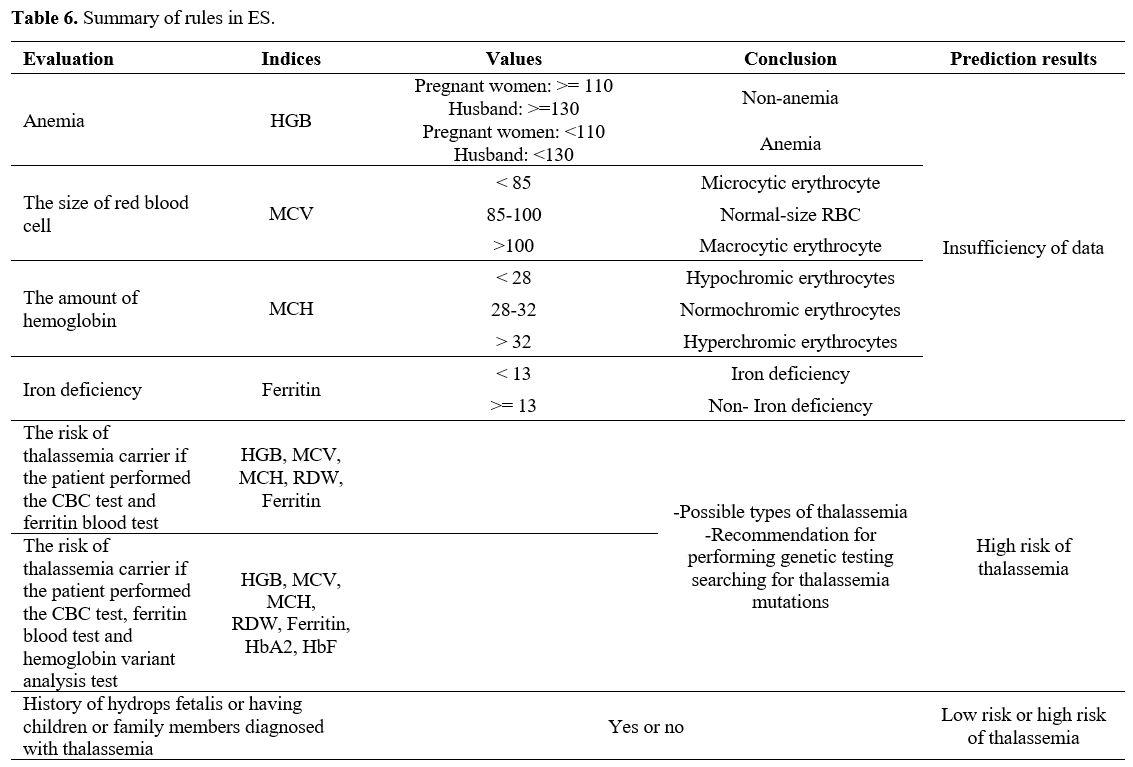 |
Table 6. Summary of rules in ES.
|
 |
Table 7. Evaluation of the effectiveness of the expert system (ES).
|
 |
Table 8. Comparison between the expert system and AI model when
using only the 4 most important features in general CBC, HPLC and iron
status database (pregnant women and their husbands).
|
Discussion
The
present study analyzed the prevalence of different thalassemia types
among pregnant women and their husbands who came to the Vietnam
National Hospital of Obstetrics and Gynecology. The prevalence of
different thalassemia types in fetuses of parents with established,
according to PCR tests, thalassemia genes was also evaluated. The
prevalence of alpha-thalassemia in pregnant women, husbands, and
fetuses was higher than in beta-thalassemia carriers. (Tables 1, 2).
The
prevalence may have been because women whose babies had hydrop details
in the previous pregnancy or the fetuses with alpha thalassemia would
show clinical manifestations, so they would go for a check-up and
perform prenatal screening tests to do the treatment or prevent
thalassemia for the next pregnancy. The same reason could explain why
the prevalence of alpha-thalassemia carriers of pregnant women who were
screened for thalassemia was also higher than beta-thalassemia at the
prenatal diagnostic center in Central Obstetrics Hospital in the study
conducted by Dang.[22]
In our study, 8841
pregnant women were screened by CBC, HPLC, and iron status test; the
prevalence of alpha-thalassemia carriers was 6.4%, the prevalence of
beta-thalassemia carriers was 1.32%, the prevalence of co-inheritance
of alpha and beta-thalassemia was 0.08% (Table 1),
which were higher than those studied by Nguyen Khac Han Hoan. In this
study, the percentages of pregnant women carrying the alpha-thalassemia
carriers, beta-thalassemia carriers, and co-inheritance of alpha and
beta-thalassemia were 1.11%, 0.52%, and 0.07%, respectively. However,
in both studies, the percentage of pregnant women and pregnant women
with alpha-thalassemia carriers was the highest (6.4%, 61.85%, and
1.11%, 71.3%).[23] However, our results were lower compared to the research of Sheng He et al. (8.1% and 3.4%)[24] and Naili Husna's study (alpha gene carrier thalassemia is 9.8%).[25] The difference in size, region, and country can explain the reason for these differences.
Nowadays,
one of the most important components in family planning and pregnancy
is detecting and preventing hereditary pathologies in future offspring.
In this regard, genetic tests and consultations are conducted for
future parents, based on which final decisions are made. The clinical
decision support system (CDSS), which is currently being widely
implemented in various fields of medicine, can undoubtedly provide
significant assistance to physicians in the diagnosis and prognosis of
hereditary pathologies.[26]
Thalassemia is a
complicated disease without a cure, excluding allogeneic hematopoietic
stem cell transplantation. Currently, the best strategies for
thalassemia management are screening for thalassemia based on family
history, cell blood count, serum iron, and ferritin. However, this is a
challenge for primary health care facilities and ethnic minority areas
due to the limited understanding of thalassemia not only by the
population but also by the grassroots medical staff. To solve this
problem, the authors conducted a clinical decision support system to
support physicians in screening and diagnosing thalassemia. The
clinical decision support system (CDSS) is a system comprising 2
different systems: The expert system (ES) and the AI-based clinical
decision support system (AI-based CDSS).
The Expert system is a
knowledge-based clinical decision support system encoding the experts'
knowledge into an automated system.[27] The operating
principle of the ES is the simulation of the procedure of diagnosis and
screening for thalassemia done by medical physicians. It aims to
support doctors dealing with complicated cases, especially at the
commune health centers facing a shortage of qualified health workers.
Like other expert systems, it comprises three main parts: The knowledge
base, the inference engine, and the EHR front-end interface. The
knowledge base includes a set of if-then rules built based on red blood
cell indices, ferritin, and hemoglobin electrophoresis results using
the guideline for prenatal screening for thalassemia of WHO and the
Vietnam Ministry of Health (Table 8).
The
guideline comprises recommendations proposed by experts and used by
medical physicians to screen and diagnose thalassemia in clinical
practice; thus, it is reliable and suitable for the racial
characteristics of Vietnamese people. The system also includes a
knowledge update interface, with which the experts can enrich the
knowledge base by directly updating their clinical experience and new
medical knowledge. This part is important to ensure the accuracy and
the update of the expert system because the knowledge of thalassemia
can be updated and changed over time. The inference engine is an
essential part of the expert system, which applies the if-then rules of
the knowledge base to the patient's clinical data to create an
inference. Development of the inference engine is an important step
when building an expert system to increase the system's accuracy in the
research; the three-layer model was applied.
The EHR front-end interface of the model has 2 sections: the input section and the output section (Figure 3).
The input section includes 9 boxes to enter patients' clinical
information, including cell blood count, plasma iron, and ferritin. The
output section, which includes 3 boxes, shows the conclusion drawn by
the inference engine. The conclusions drawn by the expert system
predict the risk of thalassemia, whether it is high risk or low risk,
and the physicians can identify if this case requires diagnostic
genetic testing.
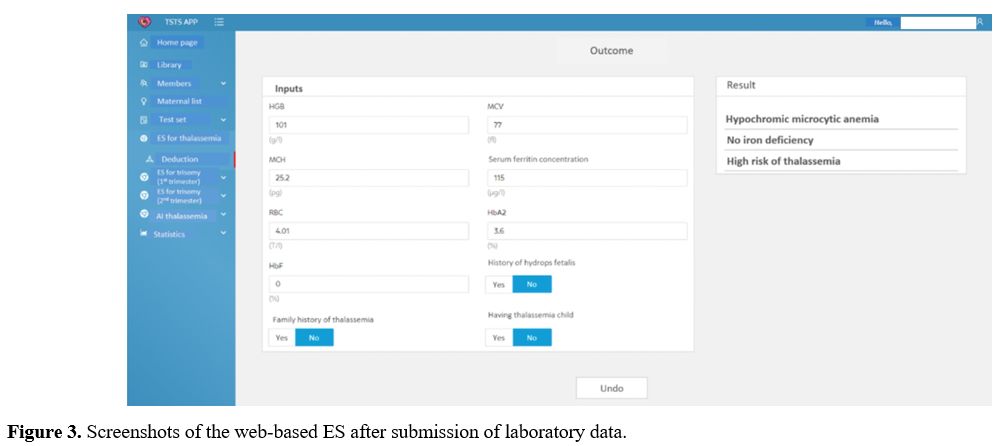 |
- Figure 3. Screenshots of the web-based ES after submission of laboratory data.
|
The
advantage of the expert system is that it is possible to explain how
the system makes the recommendation, and due to this, it has high
reliability and accuracy. Another advantage of our ES is that it is
deployed on the Internet; thus, users can access the ES on any computer
and at any time with an internet connection. The evaluation of the
effectiveness of the developed expert system (ES) in thalassemia
screening in the present study showed a high accuracy of 98.45%.
Unlike
the ES, The AI-based clinical decision support system does not use the
knowledge base. Instead, it uses a form of AI called machine learning
(ML), which allows computers to learn from past experiences and/or find
patterns in clinical data to make decisions; thus, it does not require
writing rules. Machine learning describes the computer algorithms to
determine patterns in very large datasets. Over the past years, ML has
been applied in a wide range of medical fields and demonstrated
impressive results, especially in clinical decision support, patient
monitoring, and management.[28] Many ML techniques
have been applied in building CDSS, in which K-nearest neighbors,
Support vector machine, Random Forest, and Multilayer perceptron (MLP)
are the most common techniques.[29]
To optimize
the clinical decision support system (CDSS), before constructing the
CDSS, the authors conducted to determine the features of the CBC, HPLC,
and iron status needed to screen for thalassemia. After using MID and
MDA algorithms found that 4 parameters, including HGB, MCV, MCH, and
RDW, were the most important ones in screening for thalassemia from the
database containing over 10000 cases having CBC, HPLC, and iron status
results. This report is consistent with the recommendation of WHO in
screening for thalassemia; HGB, MCV, and MCH are the parameters
currently used in screening for thalassemia in clinical practice.
In the research, the authors built an AI-based CDSS, including the AI algorithm and the EHR front-end interface.
To
determine the most appropriate AI algorithm for constructing AI-based
CDSS, the authors conducted to train the dataset on 4 models, SVM, KNN,
MLP, and RF, then evaluated them on 4 indices: accuracy, precision,
recall, and F1-score to choose the most appropriate one.
The
obtained result showed that the MLP model was the most stable
regardless of the training database. Particularly, when training with
the general CBC and iron status database for 4 most important features,
it had an accuracy of 97%, a precision of 92.68%, a recall of 99.23%,
an F1 score of 96.20% for original data, and an accuracy of 94.5%, a
precision of 88.24%, a recall of 98.68% and an F1-score of 93.17% for
SMOTE. When training with the database of pregnant women, it had an
accuracy of 97.81%, a precision of 93.21%, a recall of 99.84%, an
F1-score of 95.72% for original data, and an accuracy of 97.65%, a
precision of 93.28%, recall of 99.73%, F1-score of 96.43% for SMOTE.
Especially with only 4 important features selected above, the model's
results were extremely impressive since the accuracy of MLP increased
from 96.91% to 97.81%. Another remarkable thing about this database is
that after balancing data with SMOTE, the results had a significant
difference between the models. Thus, it is possible to temporarily
conclude that with only the data of the pregnant woman, the model is no
longer confounded by the cell blood count data of the husband. When
training with the database of husbands, it had an accuracy of 97.44%, a
precision of 96.42%, a recall of 100%, and an F1 score of 97.62% for
original data and an accuracy of 94.18%, a precision of 95.44%, a
recall of 95.95% and an F1-score of 95.18% for SMOTE.
With such
high accuracy, it is possible to apply MLP to construct an AI-based
CDSS to predict the risk of thalassemia. In fact, this has been proven
by previous research. Al Agha et al.[30] proposed an
AI model for thalassemia prenatal screening built by training and
evaluating 3 models, including KNN, NB (Navies Bayes), and MLP, among
which the MLP model got the highest accuracy with 99.73%. Egejuru et
al.[31] proposed to apply MLP to build an AI model
for thalassemia classification. In the research, the accuracy of the
MLP was 98.11%. Like the ES, The EHR fronted interference of the
AI-based CDSS has two sections: An input section and an output section (Figure 4).
The difference between the two systems is that the ES concludes the
type of anemia and whether patients are at high risk or low risk, while
the AI-based predicts the risk of thalassemia in the percentage form.
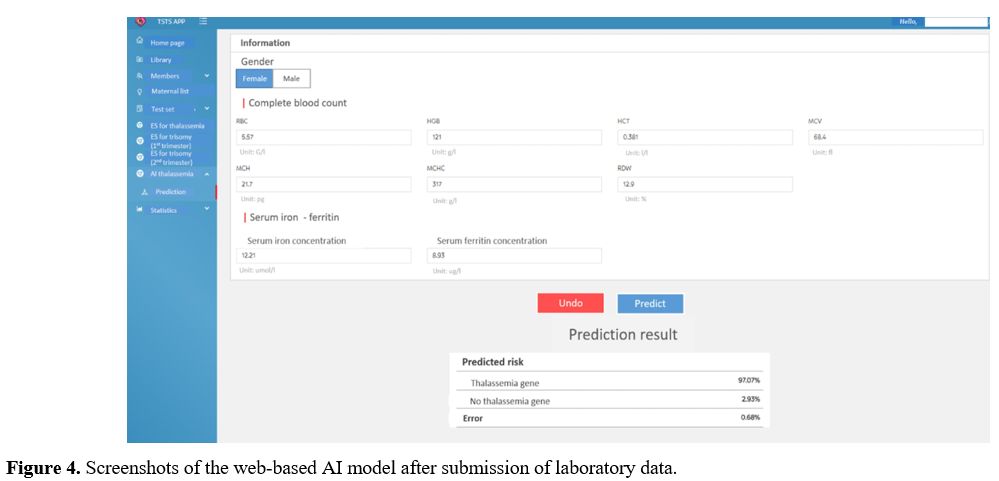 |
- Figure 4. Screenshots of the web-based AI model after submission of laboratory data.
|
Despite
its advantage, the adoption of AI in medicine is rife with challenges,
including the impossibility of explaining the logic that ML uses to
make an inference (black box). In AI-based CDSS, the users and
researchers can only know the inputs and outputs, but it is challenging
to understand how it works inside. Therefore, the accuracy of the
AI-based CDSS is questionable.[17] To deal with this
problem, the authors compared it to the ES by testing both ES and
AI-based CDSS with 396 cases of thalassemia. The result showed that
when testing on the same dataset, the AI-based CDSS got an accuracy of
94.5%, 96%, and 97% (depending on the algorithm used) when using four
important features slightly lower than ES with 98.45%. Thus, it is
possible to apply the AI-based CDSS in screening for thalassemia.
However, it should be noted that the proposed AI-based CDSS for
thalassemia screening is experimental. The advantage of algorithms
built on deep machine learning over physician-based assessments
requires more in-depth and comprehensive research. According to a
meta-analysis of publications on the use of AI in CDSS models for
various diseases, no advantage of algorithms built on deep machine
learning over physician estimates was noted.[32] It
is noteworthy that the effectiveness and safety of AI-based CDSS vary
and are ambiguous: there are both successes and failures.[33]
Regarding the proposed AI-based CDSSs, it is important to note that
future advances in genetic diagnosis of thalassemia may require a
significant revision of these CDSS and new studies to confirm the
effectiveness and safety of such systems. AI-based CDSSs are an
emerging but understudied field, requiring much effort before showing
real progress.
Conclusions
Based
on PCR tests, it was found that among pregnant women and their husbands
who came to Vietnam National Hospital of Obstetrics and Gynecology, the
rate of patients with Alpha thalassemia was 10.73% (1085 patients), the
rate of patients with beta-thalassemia is 2.24% (227 patients), and
0.29% (29 patients) of patients carry both alpha-thalassemia and
beta-thalassemia gene mutations. The authors successfully built expert
and 4 AI-based CDSS for prenatal screening for thalassemia. The expert
system developed based on WHO, and Vietnamese Ministry of Health rules
and guidelines for prenatal thalassemia screening showed an accuracy of
98.45%. Among the AI-based CDSS developed, the MLP model was the most
stable regardless of the training database (accuracy of 98,5% using all
features and 97% using only the 4 most important features). When
comparing the expert system with the AI-based CDSS, comparable accuracy
of the expert system and AI-based models was established. Thus,
AI-based CDSS showed satisfactory results. Further development of such
systems is promising with a view to their introduction into clinical
practice.
References
- Sheth S, Thein SL. Thalassemia: a
disorder of
globin synthesis. In: Kaushansky K, Prchal JT, Burns LJ, Lichtman MA,
Levi M, Linch DC, eds. Williams Hematology 10, New York, McGraw-Hill
Education. 2021; 12-13.
- Gell DA. Structure and function
of haemoglobins. Blood Cells Mol Dis. 2018;70:13-42. https://doi.org/10.1016/j.bcmd.2017.10.006
PMid:29126700
- Viprakasit
V, Ekwattanakit S. Clinical classification, screening and diagnosis for
thalassemia. Hematol Oncol Clin North Am. 2018;32:193-211. https://doi.org/10.1016/j.hoc.2017.11.006
PMid:29458726
- Petrakos
G, Andriopoulos P, Tsironi M. Pregnancy in women with thalassemia:
challenges and solutions. Int J Womens Health. 2016;8:441-51. https://doi.org/10.2147/IJWH.S89308
PMid:27660493 PMCid:PMC5019437
- Shang
X, Xu X. Update in the genetics of thalassemia: what clinicians need to
know. Best Pract Res Clin Obstet Gynaecol. 2017;39:3-15. https://doi.org/10.1016/j.bpobgyn.2016.10.012
PMid:27876354
- Farashi
S, Harteveld CL. Molecular basis of α-thalassemia. Blood Cells Mol Dis.
2018;70:43-53. https://doi.org/10.1016/j.bcmd.2017.09.004
PMid:29032940
- Shah FT, Sayani F, Trompeter S, Drasar E,
Piga A. Challenges of blood transfusions in β-thalassemia. Blood Rev.
2019;37:100588. https://doi.org/10.1016/j.blre.2019.100588
PMid:31324412
- Bain BJ. Delta beta thalassemia. Am J Hematol.
2020;95:116. https://doi.org/10.1002/ajh.25623
PMid:31444804
- Borgna-Pignatti
C, Gamberini MR. Complications of thalassemia major and their
treatment. Expert Rev Hematol. 2011;4:353-66. https://doi.org/10.1586/ehm.11.29
PMid:21668399
- Thalassaemia
International Federation. [Internet]. Response to the proposal for the
inclusion of whole blood and red blood cells on The WHO Essential
Medicines Lists (EML). 2013. https://issuu.com/internationalthalassaemiafederation/docs/tif_magazine_issue_62
(accessed 15 December 2022)
- Fucharoen S, Winichagoon P. Haemoglobinopathies in
southeast Asia. Indian J Med Res. 2011;134:498-506.
- Nguyen KHH. Thalassemia and a model of prevention
in Vietnam. Sydney: The University of Sydney; 2005.
- Dossanova
A, Lozovoy V, Wood D, Lozovaya Y. Reducing the risk of postoperative
genital complications in male adolescents. Int J Environ Sci Educ.
2016;11:5797-807.
- Thalassaemia International
Federation. Guidelines for the Management of Transfusion Dependent
Thalassaemia; 2021. https://thalassaemia.org.cy/publications/tif-publications/guidelines-for-the-management-of-transfusion-dependent-thalassaemia-4th-edition-2021/
(accessed 15 December 2022)
- Dossanov B, Trofimchuk
V, Lozovoy V, Khmyzov S, Dossanova A, Zhukenov O, Tuktiyeva N, Angelov
A. Evaluating the results of long tubular bone distraction with an
advanced rod monolateral external fixator for achondroplasia. Sci Rep.
2021;11:14727. https://doi.org/10.1038/s41598-021-94146-z
PMid:34282216 PMCid:PMC8290032
- Kawamoto K, Houlihan CA,
Balas EA, Lobach DF. Improving clinical practice using clinical
decision support systems: a systematic review of trials to identify
features critical to success. BMJ. 2005;330:765. https://doi.org/10.1136/bmj.38398.500764.8F
PMid:15767266
PMCid:PMC555881
- Sutton RT, Pincock D, Baumgart DC,
Sadowski DC, Fedorak RN, Kroeker KI. An overview of clinical decision
support systems: benefits, risks, and strategies for success. NPJ Digit
Med. 2020;3:1-10. https://doi.org/10.1038/s41746-020-0221-y
PMid:32047862 PMCid:PMC7005290
- Muhiyaddin R,
Abd-Alrazaq AA, Househ M, Alam T, Shah Z. The impact of clinical
decision support systems (CDSS) on physicians: a scoping review. Stud
Health Technol Inform. 2020;470-3.
- Amendolia SR,
Brunetti A, Carta P, Cossu G, Ganadu ML, Golosio B, Mura GM, Pirastru
MG. A real-time classification system of thalassemic pathologies based
on artificial neural networks. Med Decis Making. 2002;22:18-26. https://doi.org/10.1177/0272989X0202200102
PMid:11833662
- Masala
GL, Golosio B, Cutzu R, Pola R. A two-layered classifier based on the
radial basis function for the screening of thalassaemia. Comput Biol
Med. 2013;43:1724-31. https://doi.org/10.1016/j.compbiomed.2013.08.020 PMid:24209918
- Chawla
NV, Bowyer KW, Hall LO, Kegelmeyer WP. SMOTE: synthetic minority
over-sampling technique. J Artif Int Res. 2020;16:321-57. https://doi.org/10.1613/jair.953
- Dang HTT.
Screening for Thalassemia in Pregnant Women Who Come for Examination
and Treatment at the National Hospital of Obstetrics and Gynecology.
Vietnam, National Hospital of Obstetrics and Gynecology. 2019.
- Nguyen
HHK. Research for Alpha and Beta Thalassemia Screening and Diagnosis.
Vietnam, Ho Chi Minh City University of Medicine and Pharmacy. 2013.
- Sheng
H, Qiang Z, Dongming L, Chen S, Tang Y, Chen Q, Zheng C. Prevention and
control of Hb Barts disease in Guangxi Zhuang Autonomous Region, China.
Eur J Obstet Gynecol. 2020:178:138-41. https://doi.org/10.1016/j.ejogrb.2014.03.034
PMid:24792538
- Husna
N, Handayani NSN. Molecular and haematological characteristics of
alpha-thalassemia deletions in Yogyakarta special region, Indonesia.
Rep Biochem Mol Biol.
2021;10:346-53. https://doi.org/10.52547/rbmb.10.3.346
PMid:34981010 PMCid:PMC8718782
- Sarafidis M, Manta O,
Kouris I, Schlee W, Kikidis D, Vellidou E, Koutsouris D. Why a clinical
decision support system is needed for tinnitus? Annu Int Conf IEEE Eng
Med Biol Soc.
2021;2021:2075-8. https://doi.org/10.1109/EMBC46164.2021.9630137
PMid:34891697
- Klar R, Zaiß A. Medical expert systems:
design and applications in pulmonary medicine. Lung. 1990;168:1201-9.
https://doi.org/10.1007/BF02718262 PMid:2117122
- Rowe M.
An introduction to machine learning for clinicians. Acad Med.
2019;94:1433-6. https://doi.org/10.1097/ACM.0000000000002792 PMid:31094727
- Shaikh
F, Dehmeshki J, Bisdas S, Roettger-Dupont D, Kubassova O, Aziz M, Awan
O. Artificial intelligence-based clinical decision support systems
using advanced medical imaging and radiomics. Curr Probl Diagn Radiol.
2021;50:262-7. https://doi.org/10.1067/j.cpradiol.2020.05.006
PMid:32591104
- AlAgha AS, Faris H, Hammo BH, Ala'M AZ.
Identifying β-thalassemia carriers using a data mining approach: the
case of the Gaza Strip, Palestine. Artif Intell Med. 2018;88:70-83. https://doi.org/10.1016/j.artmed.2018.04.009
PMid:29730048
- Egejuru
NC, Olusanya SO, Asinobi AO, Adeyemi OJ, Adebayo VO, Idowu PA. Using
data mining algorithms for thalassemia risk prediction. Int J Biomed
Sci Eng. 2019;7:33. https://doi.org/10.11648/j.ijbse.20190702.12
- Liu
X, Faes L, Kale AU, Wagner SW, Fu DJ, Bruynseels A, Mahendiran T,
Moraes G, Shamdas M, Kern C, Ledsam JR, Schmid MK, Balaskas K, Topol
EJ, Bachmann LM, Keane PA, Denniston AK. A comparison of deep learning
performance against health-care professionals in detecting diseases
from medical imaging: a systematic review and meta-analysis. The Lancet
Digital Health.
2019;1:e271-97. https://doi.org/10.1016/S2589-7500(19)30123-2
PMid:33323251
- Roshanov PS, Fernandes N, Wilczynski JM,
Hemens BJ, You JJ, Handler SM, Nieuwlaat R, Souza NM, Beyene J, Van
Spall HGC, Garg AX. Features of effective computerised clinical
decision support systems: metaregression of 162 randomised trials. BMJ.
2013;346:f657. https://doi.org/10.1136/bmj.f657
PMid:23412440
[TOP]